Introduction and motivation
Eric Schmidt famously stated that “the Internet will disappear” given that there will be so many things that we are wearing and interacting with that we won’t even sense the Internet, though it will be part of our presence all the time. Although this first might sound a bit surprising, it is actually what profound technologies do in general. In “The Computer for the 21st Century,” Mark Weiser argued that the most profound technologies are those that disappear. They weave themselves into the fabric of everyday life until they are indistinguishable from it.
An interesting approach to make the Internet disappear is the so-called Naked World vision that aims at paving the way to an Internet of No Things. The term Internet of No Thing s was coined by Demos Helsinki founder Roope Mokka in 2015. The term means that a user can live without having to carry gadgets (i.e., naked), but is able to access digital services when needed and after that, the user interface of the services will disappear [1].
The Internet of No Things will evolve in three different phases. The first phase involves today’s bearables. In this phase, a user is able to access digital services through components like tablets, PCs, smartwatches, smartphones, etc. The second phase involves emerging wearables. In this phase, users are able to access digital services through tools like smart watches, smart jackets, etc. The final phase will be so-called nearables. In this phase, the environment around us is equipped with smart components that are distributed and communicate with each other. The concept of nearables is very close to ubiquitous and pervasive computing. This type of computing leverages on distributed computing, in which a user is able to access the desired service based on the environment and her location, history, and current situation. Current computation methods should evolve to be able to support the envisioned Naked world’s requirements.
Extended Reality (XR)
Extended reality (XR) is the next-generation computing platform that will change our life significantly. XR enables human-machine interaction that will help us change our daily communication in real-life [2]. Importantly, XR creates a bridge between our real world and the digital world. As shown in Fig. 1, XR acts as an umbrella term that encompasses Augmented Reality (AR), Virtual Reality (VR), and Mixed Reality (MR). Note that while AR integrates virtual and real objects in real-time, VR allows users to control and navigate their movements in a simulated world.
There are many examples of extended reality, e.g., playing games, traveling through the universe, walking through 3D models of buildings, virtual tours, etc. This technology also has many applications in vast areas like brain injury rehabilitation, automobile design, tourism, education, retailing, healthcare, among others. By integrating VR and AR we can realize the benefits of mixed reality (MR). MR allows us to create new environments and visualizations in that physical and virtual objects interact with each other in real-time (e.g., Microsoft's HoloLens). One of the challenges of XR is collecting and processing huge amounts of detailed and personal data about what people look at, their emotions, and other personal information.
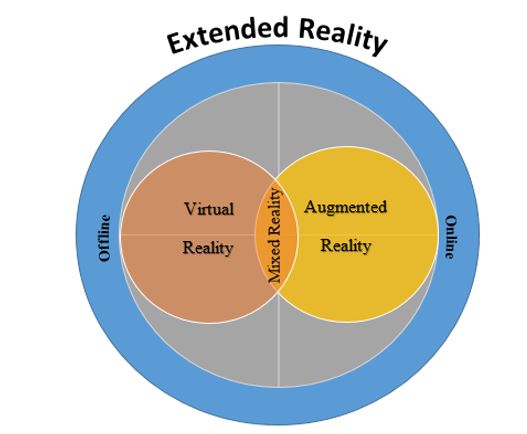
Fig. 1. XR encompassing virtual, mixed, and augmented reality.
Another challenge is implementation cost. For instance, to implement XR we need wearable devices that allow for a full XR experience and also computers that have suitable resources regarding hardware, display, power, and connectivity. 6G holds promise to address these challenges [3].
Ubiquitous/Pervasive/Persuasive Computing
Another approach towards making computation invisible is the concept of ubiquitous and pervasive computing [2]. In this concept, computation has the capability to obtain information from the environment, in which it is embedded, and utilize it to dynamically build computing models. Furthermore, it has the capability to detect other computing devices entering it. A user’s access to related and localized services is limited such that useful services prevent the user from exploiting the computing resources of their environment. The main challenge in ubiquitous computing is integrating large-scale mobility with pervasive computing functionality.
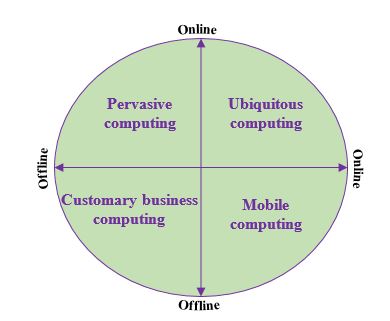
Fig. 2. Comparison of different types of computation.
As shown in Fig. 2, ubiquitous computing refers to any computing device, which is able to move with us, create a dynamic model of various environments, and configure its service based on given demands and locations. Furthermore, the involved computing devices are able to remember the past environment they operated in, thus helping users work when they re-enter or and activate services in the new environment.
Fig. 3 illustrates the generic Internet of No Things architecture integrating bearables, wearables, nearables, and the aforementioned different types of computation. In this architecture, we use the latest humanoid robot (Software's so-called Pepper) as a nearable. More details about Pepper will be provided below in the social robot section. For implementing ubiquitous computing, we have to tackle various challenges. We have to find new mechanisms for the design and implementation of specific architectures that can be reconfigured. We also have to rethink feasible architectures, design ontologies, domain models, requirements, and interaction scenarios. Another important challenge is the interplay between technical and social issues. In particular, social trust is an important issue.
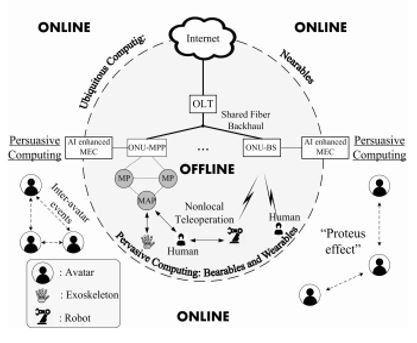
Fig. 3. Generic architecture of the Internet of No Things integrating bearables, wearables, and nearables [2].
Ubiquitous computing may be viewed as the third era in computing, of which we are just at the beginning. The first era was mainframe computers, each shared by many users. Next came the personal computing era, where each person had her own personal computers, smart watch, tablet, smartphone, etc. The third era is ubiquitous computing, where technology moves into the background of our real-life environments. In this era, embedded computers interact with the environment regarding both physical and social aspects. The main idea is about transitioning to a more distributed, mobile, and embedded form of computing tools. Over the last few years, there has been significant progress been made towards achieving these goals. Hardware can be produced on small scale with the same computing capacity as large-scale counterparts at smaller power consumption and cheaper production cost. To unleash the full potential of ubiquitous and pervasive computing, both need to be integrated. Although these terms are used most of the time interchangeably, they are conceptually different. Ubiquitous computing increases our capability to move computing services physically. In other words, ubiquitous computing enables us to access computation resources regardless of our location. Towards this end, the physical size of computers needs to be significantly increased. Furthermore, context awareness will be instrumental in realizing the benefits of ubiquitous computing.
Social Robots
Human-Robot Interaction (HRI)
Due to the rapidly increasing human-robot interactions (HRI) in our everyday life and in industry, a proper understanding of the concept behind this term is important. HRI is a field of study dedicated to understanding, designing, and evaluating robotics systems for use by or with humans [4]. The term interaction implies communications between robots and humans that may have different forms. The communications underlying HRI may be categorized into remote interaction and proximate interaction. In remote interaction, the human and robot are not at the same location.
Hence, a communications network is needed to facilitate remote communications. For instance, today we may use cloud technology that creates a very easy and user-friendly communications infrastructure. Conversely, in proximate interaction, the human and robot are at the same location. HRI becomes interesting in this type of interaction since social behavior comes into play. In proximate interaction, researcher studies consider aspects such as empathy, emotions, behavior, cognition, physical interactions, and also social norms.
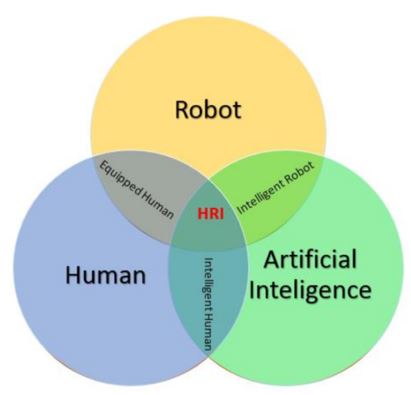
Fig. 4. HRI research fields of interest.
Fig. 4 illustrates the three most important research fields of interest of HRI as intersecting circles. The first circle shows the robot. According to Wikipedia, “a robot is a machine, especially one programmable by a computer that is capable of carrying out a complex series of actions automatically.” Clearly, this is a rather simple definition of a robot. In general, it is not easy to define what robots are and categorize them, because each robot has its own specific features. Generally, robots are categorized based on their respective usage scenario:
- Aerospace
- Consumer
- Rescue robot
- Drone
- Education
- Entertainment
- Exoskeleton
- Humanoid and social robot
- Industrial
- Medical
- Military and security
In our research, we focus on social robots since this they are expected to have a high impact on the future of HRI. Social robots include humanoid robots such as the aforementioned Pepper as well as Software's Nao and Honda’s Asimo, among others. In the next section, we describe Pepper in technically greater detail. The second circle of Fig. 4 involves the human. A human is a person who interacts with a robot ideally via peer-to-peer communications. It is important to note that in our research the human and robot play complementary roles. In our research, we intend that robots and humans learn from each other. The third circle is artificial intelligence (AI). AI helps humans and robots work with each other more easily. For instance, a robot's gestures may be improved based on human gestures by means of AI. The intersection of robot and AI renders an intelligent robot, whereby the robot’s behavior is enhancing the same as human behavior. Another interesting intersection is that of AI and human, which renders humans more intelligent. Humans may use AI as a tool to do perform daily tasks with higher efficacy and accuracy. The last intersection is between human and robot. For instance, a human may use the robot in locations that are dangerous for humans such as buildings on fire, environments with chemical gases, or military environments. As shown in Fig. 4, the intersection of all of the three circles is where HRI lies.
Robotic/Embodied Communication
During face-to-face communications, humans do not exchange just oral information. They also use their body to transfer information to their peers. Most of the time communications takes place in a shared context, where peers jointly attend and have common knowledge about the subject. The first research studies on gestures as a mechanism of communications were done around 1980. McNeil was the first person to study so-called embodied communications. He found that gestures are synchronized with speech and both are closely connected in the cognitive system. The elements of embodied communication include eye contact, facial expression, body gesture, head movement, etc.
Although the number of social robots is constantly rising, most of them are not yet fully involved in humans' life. For instance, while Google's assistant is able to answer questions in multiple languages and Amazon's Alexa witnesses the presence of home robots, for social robots the scenario is different. The aforementioned concept of embodied communications is more significant for social robots since they interact with humans. To do so, social robots use nonphysical tools that help them have effective social interactions. Embodiment enables the robot to have more channels of communications. These communication channels also render the robot more trustworthy.
Humanoid Robot “Pepper”
Pepper is the next generation of Aldebaran's NAO robot. Without any doubt, we can say that currently Pepper is the most powerful and popular humanoid robot. Although NAO filled the gap of HRI, Pepper brings HRI to a new level by providing novel functionalities. Pepper is an autonomous humanoid robot designed by Aldebaran Robotics. In 2015, Pepper was released by SoftBank. The main target of Pepper is social interaction with humans. Its height and physical appearance render Pepper more similar to humans than NAO did. The main role of Pepper is the engagement of people. It is one of the best choices for researchers working on HRI. Pepper uses a software called “Choregraphe,” which makes the work of programmers easy by providing good interface and pre-defined libraries.
As shown in Fig. 5, Pepper's ecosystem comprises humans, VR goggles, other virtual/physical robots such as NAO, and cloud computing API tools, most notably, Google Cloud, Microsoft Azure, and IBM Watson. In this ecosystem, human interact with a robot ideally through peer-to-peer communication. It’s important to note that in this ecosystem humans and robots assume a complementary role, each with its own respective requirements. Adding VR to Pepper’s ecosystem is a promising approach for enhancing human-robot interaction. For instance, VR based teleoperation provides a human with the possibility of visualizing the robot's perspective. By adding other virtual and physical robots to Pepper’s ecosystem, the performance and capabilities of Pepper will increase by performing a variety of complex tasks. Among others, it is possible that Pepper invites NAO for collaborating and carrying certain actions, which Pepper wouldn't be able to accomplish completely on his own. Furthermore, virtual robots are useful in situations, which don't allow for testing a given scenario through real robots. In such situations, virtual robots are suitable tools to save costs and time. Similarly, cloud computing has a beneficial impact on Pepper’s ecosystem. Cloud computing provides Pepper with on-demand access to parallel grid computing for purposes such as statistical analyzing, learning processes, and motion planning. By using cloud computing, we can improve the HRI performance significantly. For instance, IBM Watson has an API for analyzing the tone of human conversation. This tool makes use of a linguistic analyzer for detecting both emotion and tone within written text. The service is able to analyze the tone at the document and sentence level. By using the AI service, Pepper is able to extract the tone in text-based conversations in order to add a sense of empathy to HRI. Currently, Pepper's latest version is 2.5.10. This version offers major upgrades of critical components such as APIs, software, and tablets. Pepper has a weight of 28 Kg/62 Lb and 120cm/4 feet tall. The operating system is Linux-based NAOqi. This operating system contains several libraries, which enable developers to control the robot’s resources, as depicted in Fig. 6.
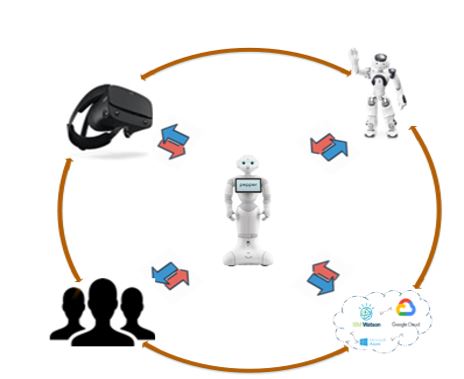
Fig. 5. Pepper’s ecosystem.
The ground on which Pepper moves should be smooth and Pepper is intended for indoor use due to the installed laser sensors that require a finite distance to a barrier. With a fully charged battery, Pepper runs up to 8 hours, depending on the intensity of the performed tasks. Pepper may be charged during operation via power cables. The processor of Pepper is an ATOM E3854, 1.9 GHz quad-core with 1 GM RAM and 2 GB Flash. Pepper’s tablet is full HD with 1GB memory. Pepper communicates wirelessly via WiFi 802.11a/b/g/n or alternatively may use an Ethernet cable.
There are many ways to realize communications between a human and Pepper by using his touch sensors (on Pepper’s head, palms, and arms), 2D and 3D cameras, tablet, and speech recognition. The tablet enables Pepper to show images, videos, and web pages. All these represent are human-robot interaction channels. In addition, Pepper is equipped with three omnidirectional wheels, which let Pepper move 360 degrees. The speed of Pepper robot is limited by 3 km/h. Each of Pepper’s LEDs has a different color with a specific meaning. The most important LEDs are placed in Pepper’s eyes. When Pepper’s eyes change to blue, this means that Pepper is currently listening to the human’s voice. Developers may create different applications for Pepper using a number of different programming languages such as Python, Java, C++, C#, and Android. The communication model and exemplary methods in modules are explained in Fig. 6. The broker loads a preference stored in a file called autoload.ini that defines which libraries it will load. Each library contains one or more modules, which use the broker to advertise the methods. Developers may also use the different sensor readings and process them in order to control the servo motors.
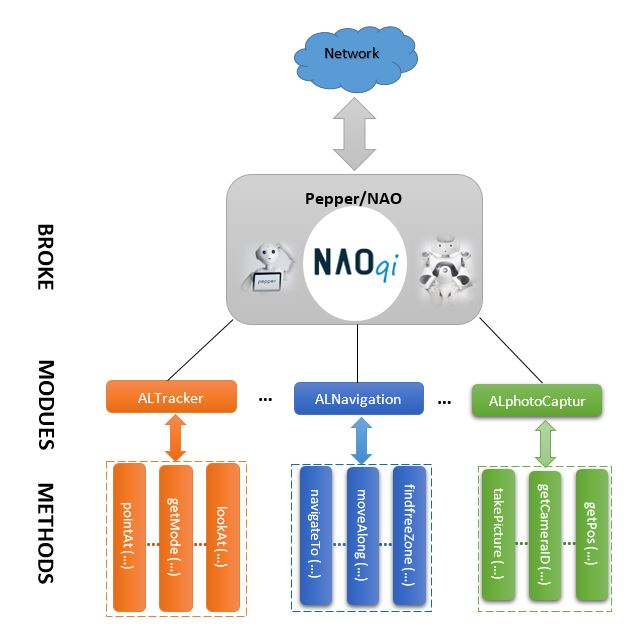
Fig.6. Pepper’s operating system NAOqi: Relation between broker, modules, and methods.
Recent progress
The research area of HRI involving Pepper has see significant progress over the last few years. In [5], the authors presented a framework to support the next generation of robots for solving problems that are not suitable to conventional programming methods. In the presented framework, Pepper uses the initial state observed from the image and the goal state learned from the human demonstration to generate an executable plan for the manipulator robot. In [6], the authors investigated the impact of the user's cultural background while generating emotional body expressions on social robots. The experimental results indicate that the proposed approach provides the robot with the capability of entering into a scenario of interaction for learning purposes. In [7], the authors studied how culture can be incorporated into a robot emotion representation model and how the emotion can be expressed physically through body movement. The proposed behaviour enables Pepper to show emotional states in various ways through a combination of valence and arousal values. The authors of [8] examined ways to let the robot imitate people's behaviour by varying the speed/exaggeration of motion and the pitch of voice. The purpose of this study was to enhance familiarity between robots and humans via imitation. The presented results show that varying the exaggeration of motion and pitch of voice affected the achievable impression significantly. In [9], the authors explored how autonomy, robustness, and error handling influence human perceptions of robot intelligence and interaction with robots. The authors suggested that robots that offer dynamically changing levels of autonomy and mitigation strategies in error situations do not negatively impact perceived intelligence and likability ratings for long-term HRI. Another study on Pepper regarding HRI was done [10], where the authors proposed an immersive, hands-free interface for Wizard of OZ (WoZ) teleoperation. The proposed system consisted of a VR headset, which connects to the robot’s camera, and a leap motion controller to enable natural real-time interaction in a WoZ style experimentation. The immersive experience provided by the VR headset together with the hands-free nature of the leap motion controller create a user-friendly and effective interface for robot teleoperation. In [11], the authors described their efforts to improve the capabilities of Pepper by adding personalized interactions to humans based on face recognition. The authors Pepper’s tablet and LEDs to realize non-verbal communications and presented a technique to simultaneously record and stream audio to a speech recognition web service in order to reduce the delay in speech interaction. In [12], the authors proposed a framework to infer the user’s personality traits during human-robot face-to-face interactions. The user’s non-verbal features were efficiently extracted from the robot’s perceptual functions during social interaction. And finally, in [13], the authors addressed the problem of human action recognition in the context of robotic platforms. The authors described a processing pipeline, which was integrated with a social robotic framework. The pipeline integrated a pose estimation module based on OpenPose, whose outputs were fed to the proposed action recognition model. The created pipeline allows applications to be built on top of the social robotic framework.
Research Directions
It may sound like a dream to design a holistic system consisting of physical robots, virtual robots, humans, XR, and cloud APIs. Most of today's researchers work on mobile robots, IoT and AI/ML separately. We think that it is time to combine these different research axes together to let emerge new possibilities. At present, collaboration and communications between robots and humans is becoming a hot topic in the research area of HRI. By fully exploiting Pepper’s ecosystems, social robots are anticipated to become more instrumental in serving more advanced services. Many cloud services exist in support of a plethora of services. For instance, IBM Watson AI, Microsoft Azure Ethereum, and Google provide a number of powerful AI services. By using Pepper as a hub connecting humans, VR goggles, NAO, and different cloud services is promising research approach, whereby each technology has its complementary role.
As a result, enhancing and evaluating the respective capabilities of humans and robots and designing a comprehensive framework for training them represents a important challenge in the HRI field. In the future, we may also need to include other research areas such as cognitive science, linguistics, psychology, and human rights aspects. According to Michael A. Goodrich, some of the HRI research challenges include but are not limited to:
- Level and behavior of autonomy
- Nature of information exchange
- Structure of team
- Adaptation, learning, and training of human and the robot
- Task shaping
Researchers
Graduate Student
Advisor
Publications
- A. Beniiche, S. Rostami, and M. Maier,
“Society 5.0: Internet as if People Mattered,”
IEEE Wireless Communications, to appear
- M. Maier, A. Ebrahimzadeh, A. Beniiche, and S. Rostami,
“The Art of 6G (TAO 6G): how to wire Society 5.0 (Invited Paper),”
IEEE/OSA Journal of Optical Communications and Networking, OFC 2021 Special Issue, vol. 14, no. 2, pp. A101-A112, Feb. 2022
- M. Maier,
“6G as if People Mattered: From Industry 4.0 toward Society 5.0 (Invited Paper),”
Proc., 30th International Conference on Computer Communications and Networks (ICCCN), Athens, Greece, July 2021
- M. Maier,
“6G Wanderlust: Toward the Internet of No Things to "See the Invisible" (Invited Keynote),”
Proc., 25th International Conference on Optical Network Design and Modelling (ONDM), Gothenburg, Sweden, June/July 2021
- M. Maier,
“Toward 6G: A New Era of Convergence (Invited Paper),”
Proc., Optical Fiber Communication Conference and Exposition (OFC), San Francisco, CA, USA, June 2021
- A. Beniiche, S. Rostami, and M. Maier,
“Robonomics in the 6G Era: Playing the Trust Game With On-Chaining Oracles and Persuasive Robots,”
IEEE Access, vol. 9, pp. 46949-46959, March 2021
- A. Ebrahimzadeh and M. Maier,
“Toward 6G: A New Era of Convergence,”
Piscataway, NJ: Wiley-IEEE Press, Jan. 2021
- M. Maier,
“The Internet of No Things: From "Connected Things" to "Connected Human Intelligence" (Invited Keynote),”
Proc., IEEE International Symposium on Networks, Computers and Communications (ISNCC), Montreal, QC, Canada, Oct. 2020
- M. Maier, A. Ebrahimzadeh, S. Rostami, and A. Beniiche,
“The Internet of No Things: Making the Internet Disappear and "See the Invisible",”
IEEE Communications Magazine, vol. 58, no. 11, pp. 76-82, Nov. 2020
- M. Maier,
“Toward the Internet of No Things: Making the Internet Disappear and `See the Invisible' (Invited Panel),”
Proc., OFC, Symposium on Emerging Network Architectures for 5G Edge Cloud and How to Achieve Ultra-low Latency and High Reliability?, San Diego, CA, USA, March 2020
References
[1]
|
I. Ahmad, T. Kumar, M. Liyanage, M. Ylianttila, T. Koskela, T. Braysy, A. Anttonen, V. Pentikinen, J.-P. Soininen, and J. Huusko, “Towards Gadget-Free Internet Services: A Roadmap of the Naked world,” Elsevier Telematics and Informatics, vol. 35, no. 1, pp. 82-92, Apr. 2018.
|
[2]
|
M. Maier and A. Ebrahimzadeh, “Toward the Internet of No Things: The Role of O2O Communications and Extended Reality,” June 2019: https://arxiv.org/abs/1906.06738
|
[3]
|
W. Saad, M. Bennis, and M. Chen, “A Vision of 6G Wireless Systems: Applications, Trends, Technologies, and Open Research Problems,” Feb. 2019: https://arXiv.org/abs/1902.10265
|
[4]
|
M. A. Goodrich and A. C. Schultz, “Human-Robot Interaction: A Survey,” Foundations and Trends in Human-Computer Interaction, vol. 1, no. 3, pp. 203-275, Jan. 2007.
|
[5]
|
A. Gardecki and M. Podpora, “Experience from the operation of the humanoid robots,” Proc., Progress in Applied Electrical Engineering (PAEE), pp. 1-6, 2017.
|
[6]
|
N. T. V. Tuyen, S. Jeong, and N. Y. Chong, “Emotional Bodily Expressions for Culturally Competent Robots through Long Term Human-Robot Interaction,” Proc., IEEE/RSJ International Conference on Intelligent Robots and Systems (IROS), 2018.
|
[7]
|
T. L. Q. Dang, N. T. V. Tuyen, S. Jeong, and N. Y. Chong, “Encoding cultures in robot emotion representation,” Proc., IEEE International Symposium on Robot and Human Interactive Communication (RO-MAN), 2017.
|
[8]
|
W. F. Hsieh, E. Sato-Shimokawara, and T. Yamaguchi, “Enhancing the familiarity for humanoid robot Pepper by adopting customizable motion,” Proc., 43rd Annual Conference of the IEEE Industrial Electronics Society (IECON), 2017.
|
[9]
|
M. Bajones, “Enabling long-term human-robot interaction through adaptive behavior coordination,” Proc., ACM/IEEE International Conference on Human-Robot Interaction (HRI), 2016.
|
[10]
|
N. Tran, J. Rands, T. Williams, “A Hands-Free Virtual-Reality Teleoperation Interface for Wizard-of-Oz Control,” Proc., 1st International Workshop on Virtual, Augmented, and Mixed Reality for HRI (VAM-HRI), 2018.
|
[11]
|
V. Pereira, T. Connell, J. Veloso, M, “Setting Up Pepper for Autonomous Navigation and Personalized Interaction with Users,” April 2017: https://arxiv.org/abs/1704.04797
|
[12]
|
Z. Shen, A. Elibol, and N. Y. Chong, “Nonverbal Behavior Cue for Recognizing Human Personality Traits in Human-Robot Social Interaction,” Proc., IEEE 4th International Conference on Advanced Robotics and Mechatronics (ICARM), pp. 402-407, 2019.
|
[13]
|
M. Nan et al., “Human Action Recognition for Social Robots,” Proc., 22nd International Conference on Control Systems and Computer Science (CSCS), pp. 675-681, 2019.
|
|